Feng Liu (he/him) -- Assistant Professor at The University of Melbourne
Home
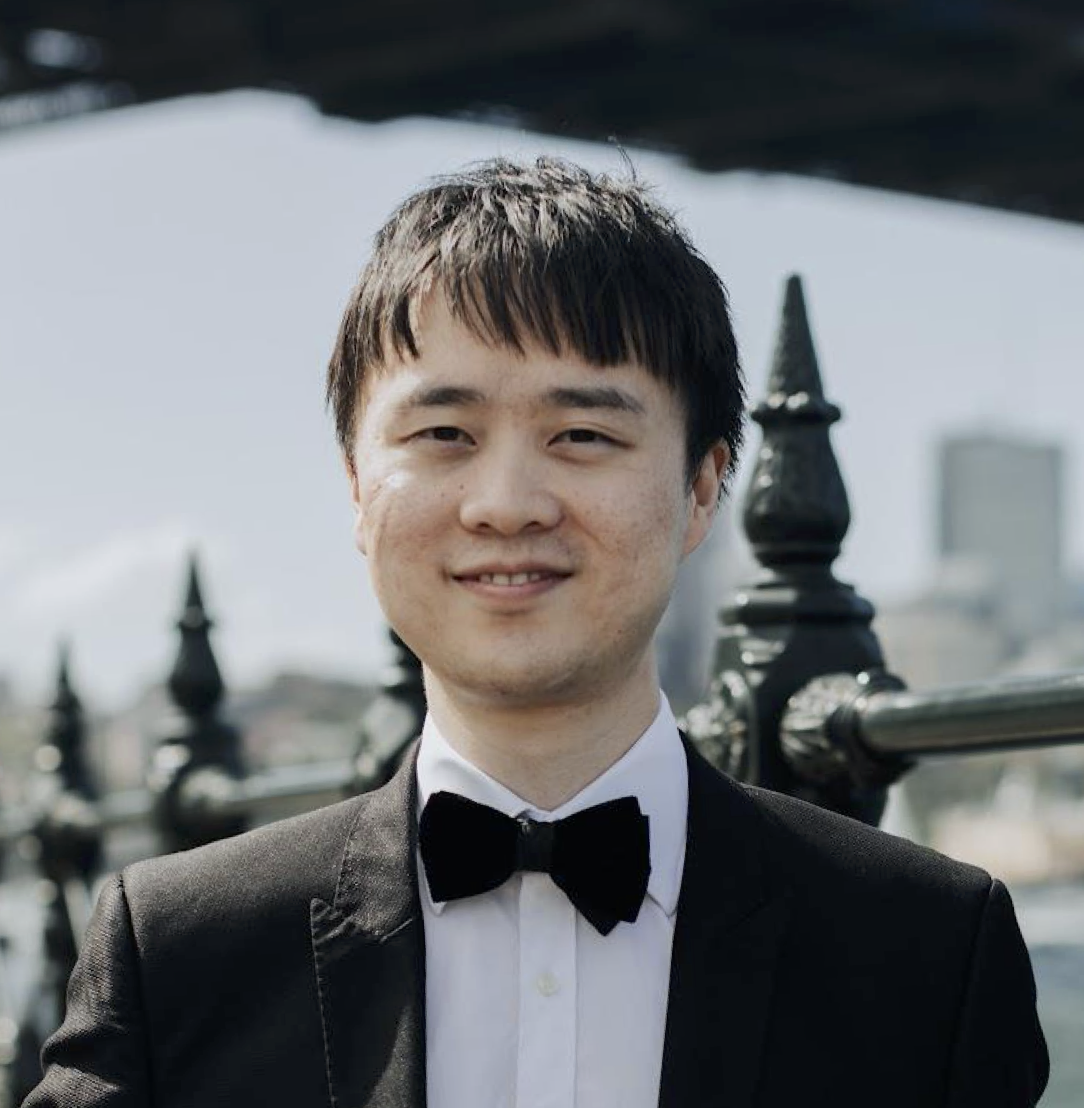 |
Feng Liu, Ph.D.
Assistant Professor in Machine Learning,
ARC Discovery Early Career Researcher Awardee (Machine Learning),
ACS Australasian AI Emerging Research Awardee,
School of Computing and Information Systems,
The University of Melbourne
Visiting Scientist @ Imperfect Information Learning Team,
RIKEN
Center for Advanced Intelligence Project (RIKEN-AIP)
Visting Fellow @ DeSI Lab,
Australian Artificial Intelligence Institute,
UTS
Address: Room 3317, Level 3, Melbourne Connect (Building 290),
700 Swanston Street, University of Melbourne VIC 3010 Australia.
E-mail: fengliu.ml [at] gmail.com or feng.liu1 [at] unimelb.edu.au
Phone: +61 3 9035 3645
[Google Scholar]
[Github]
[Group Website]
[CV]
|
Statement regarding one ICLR 2025 Submission (Feb/14/2025): We make an official statement about a factual error in one reviewer's after-discussion comments for our rejected ICLR paper regarding kernel closeness testing. This factual error is the only reason why our paper is rejected based on the meta-review (the score is changed from 8866 to 8863 40 days after the discussion deadline). Thus, it is necessary to clarify that there is no proof error in our paper, avoiding misleading future readers. Update (Mar/26/2025): We and Reviewer TXYq have reached an agreement that there is no errors and technical flaws in the theorem. Please refer to post-decision discussion under Reviewer TXYq's original comments. Meanwhile, the Area Chair also clarified that there is no proof flaws in the theorem (see the link here).
Opportunities
Long-time Recruitment (PhD): I am always looking for self-motivated PhD (two per semester). Please see this page for recruiting information, and check this page for the school information. Update (Jan. 14, 2025): No PhD position is available before July 2027.
Long-time Recruitment (RA): Meanwhile, I am happy to host remote research trainees (paid). You can collaborate with many excellent researchers in the frontier machine learning research areas in our group. Check this page for more information. Update (Aug. 15, 2024): We are actively recruiting RAs. Please send your CV to my email (Gmail).
Project-based Recruitment:
Biography
I am a machine learner with research interests in hypothesis testing and trustworthy machine learning.
I am currently an ARC DECRA Fellow and an Assistant Professor in Machine Learning at the School of Computing and Information Systems,
The University of Melbourne, Australia. We are also running the Trustworthy Machine Learning and Reasoning (TMLR) Lab where I am one of co-directors (see this page for details).
In addition, I am a Visiting Scientist at RIKEN-AIP, Japan, and a Visting Fellow at DeSI Lab, Australian Artificial Intelligence Institute, University of Technology Sydney. I was the recipient of the Australian Laureate postdoctoral fellowship. I received my Ph.D. degree in computer science at the University of Technology Sydney in 2020, advised by Dist. Prof. Jie Lu and Prof. Guangquan Zhang.
I was a research intern at the RIKEN-AIP, working on the robust domain adaptation project with Prof. Masashi Sugiyama, Dr. Gang Niu and Dr. Bo Han. I visited Gatsby Computational Neuroscience Unit at UCL and worked on the hypothesis testing project with Prof. Arthur Gretton, Dr. Danica J. Sutherland and Dr. Wenkai Xu.
I have received the ARC Discovery Early Career Researcher Award, the FEIT Excellence Award in Early Career Research at The University of Melbourne, the Outstanding Paper Award of NeurIPS (2022), the Outstanding Reviewer Award of NeurIPS (2021), the Outstanding Reviewer Award of ICLR (2021), the UTS Best Thesis Award (Dean's list), the UTS-FEIT HDR Research Excellence Award (2019) and the Best Student Paper Award of FUZZ-IEEE (2019). My publications are mainly distributed in high-quality journals or conferences, such as Nature Plants, Nature Communications, JMLR, IEEE-TPAMI, IEEE-TNNLS, IEEE-TFS, NeurIPS, ICML, ICLR, KDD, IJCAI, and AAAI. I have served as area chairs (AC) for ICML, NeurIPS, ICLR, AISTATS, senior program committee (SPC) members for AAAI, IJCAI, ECAI and program committee (PC) members for NeurIPS, ICML, ICLR, AISTATS, ACML, AAAI, IJCAI, KDD, SDM and so on. I also serve as an Editor for ACM TOPML, Associate Editor for IJMLC, Action Editor for Neural Networks, Action Editor for Transactions on Machine Learning Research and reviewers for many academic journals, such as JMLR, IEEE-TPAMI, TMLR, MLJ, and so on.
Research Highlights
May/15/2025: One paper is accepted by ACL 2025 (Findings). Congrates to the team!
May/12/2025: One paper is accepted by UAI 2025. Congrates to the team!
May/02/2025: Nine papers are accepted by ICML 2025. Congrates to the team!
Apr/29/2025: Our monograph, Trustworthy Machine Learning: From Data to Models (ISBN: 978-1-63828-548-9), is online (see pdf file here), simultaneously published in Foundations and Trends® in Privacy and Security. Congrates to the team!
Apr/05/2025: One paper is selected as CVPR 2025 highlight presentation (acceptance rate < 3.0%). Congrates to the team!
Mar/30/2025: One paper on model-inversion attack is accepted by TPAMI. Congrates to the team!
Mar/04/2025: Grateful to receive the best paper award from AAAI Colorai Workshop for our privacy-preserving low-rank Adaptation (LoRA) work published in AAAI 2025. Also grateful to secure one project from Australia's Economic Accelerator (AEA) Ignite Program, working on the AI-driven wearables for falls prevention.
Feb/17/2025: Will serve as an Area Chair for NeurIPS 2025.
Jan/23/2025: Three papers are accepted by ICLR 2025, including one paper on machine-generated text detection with deep kernel hypothesis testing, and two papers on model reprogramming (one of parameter-efficient fine-tuning methodogies). Congrates to the team!
Dec/19/2024: Grateful to receive the FEIT CIS Excellence Award in Research from the School of Computing and Information Systems at the University of Melbourne.
Nov/28/2024: Grateful to receive the Australasian AI Emerging Research Award from Australian Computer Society.
Nov/22/2024: Will serve as an Area Chair for ICML 2025.
Nov/14/2024: Will serve as an Action Editor for Transactions on Machine Learning Research.
Nov/06/2024: Grateful to receive one ARC Linkage Project. [Announcement]
Oct/24/2024: Grateful to receive the Outstanding Area Chair Award of ACM MM 2024. Welcome to Melbourne!
Oct/21/2024: Our tutorial proposal regarding model reprogramming (efficient fine-tuning) has been accepted by ACML 2024. Welcome to join ACML 2024!
Oct/03/2024: Will serve as an Area Chair for AISTATS 2025.
Sep/26/2024: Five papers are accepted by NeurIPS 2024, and one of them is selected as an oral paper (acceptance rate: 0.39%). Congrates to the team!
Sep/17/2024: Continue to be listed as one of the top 2% of the world’s most cited scientists in 2023, according to the Stanford University Report.
Aug/08/2024: Will serve as an Area Chair for ICLR 2025.
Aug/06/2024: Will serve as a senior program committee member for AAAI 2025.
Jun/13/2024: One paper is selected as ICML 2024 oral presentation (acceptance rate < 1.6%) and another one is selected as ICML 2024 spotlight (acceptance rate < 3.6%). Congrates to the team!
May/30/2024: Will serve as an Area Chair for NeurIPS 2024 Datasets and Benchmarks Track.
May/02/2024: Six papers are accepted by ICML 2024. Congrates to the team!
Apr/25/2024: Will serve as an Area Chair for NeurIPS 2024.
Apr/07/2024: One paper is accepted by JMLR. Congrates to the team!
Jan/23/2024: Will serve as an Area Chair for ICML 2024.
Jan/15/2024: One paper is accepted by ICLR 2024 and is selected as a spotlight paper (acceptance rate < 5.1%). Congrates to the team!
Jan/10/2024: One paper is accepted by Nature Communications.
Nov/27/2023: Grateful to receive the FEIT Excellence Award in Early Career Research at The University of Melbourne.
Oct/25/2023: Our tutorial proposal regarding trustworthy machine learning has been accepted by AAAI 2024. Welcome to join AAAI 2024 at the Vancouver Convention Centre in Vancouver, BC, Canada, February 20 - February 27, 2024!
Oct/04/2023: Grateful to be listed as one of the top 2% of the world’s most cited scientists in 2022, according to the Stanford University Report.
Oct/02/2023: Will serve as an Action Editor for Neural Networks.
Sep/25/2023: Our tutorial proposal regarding trustworthy machine learning has been accepted by ACML 2023. Welcome to join ACML 2023 at the Acıbadem University Conference Center in İstanbul, Turkey, November 11 - 14, 2023!
Sep/22/2023: Four papers are accepted by NeurIPS 2023. Congrats to the team!
Sep/11/2023: Will serve as an Area Chair for ICLR 2024.
Aug/25/2023: Grateful to receive ARC Discovery Early Career Researcher Award (Category: 4611 Machine Learning). [Announcement]
Jul/25/2023: Will serve as an Associate Editor for International Journal of Machine Learning and Cybernetics.
Jul/25/2023: One paper is accepted by Nature Plants.
Jul/24/2023: We will present three papers at ICML 2023 in Hawaii. Two are related to distribution-change detection (OOD detection and adversarial detection), and one is related to model adaptation.
Jul/13/2023: I officially join the School of Computing and Information Systems at The University of Melbourne as an Assistant Professor in Machine Learning (a continuing position).
Jul/10/2023: I will give a Keynote speech at the International Conference on Machine Learning and Cybernetics (ICMLC).
Jun/15/2023: Our paper regarding Responsible AI (RAI) received the ECIS Best RiP Paper Runner-up Award.
May/01/2023: We will present one paper regarding OOD detection at ICLR 2023 in Rwanda.
Mar/14/2023: Our newly proposed journal, ACM Transactions on Probabilistic Machine Learning (ACM TOPML), is officially approved! I will serve as an editor of this journal. Welcome to submit your papers to our journal!
Feb/20/2023: Grateful to secure one project from the NSF-CSIRO Joint Program in Responsible and Ethical AI. [NSF Announcement][CSIRO Announcement]
Dec/02/2022: Will serve as a senior program committee member for IJCAI 2023.
Nov/28/2022: We will present two papers at NeurIPS 2022 in New Orleans. One focuses on the learnability of OOD detection (outstanding paper), and the other uses the reprogramming property of deep neural networks to solve the OOD detection problem (spotlight paper).
Nov/24/2022: Grateful to secure one ARC Discovery Project. [Announcement]
Nov/21/2022: Our paper received the NeurIPS Outstanding Paper Award.
Nov/17/2022: One paper is accepted by Nature Communications.
Research Interests
My research interests lie in statistical hypothesis testing and trustworthy machine learning. Specifically, my current research work center around the following topics:
Statistical Hypothesis Testing:
Two-sample Testing: Testing if two datasets are drawn from the same distribution.
Goodness-of-fit Testing: Testing if data are drawn from a given distribution.
Independence Testing: Testing if two datasets are independent.
Trustworthy Machine Learning:
Defending against Adversarial Attacks: Detecting adversarial attacks (i.e., adversarial attack detection); Training a robust model against future adversarial attacks (i.e., adversarial training).
Being Aware of Out-of-distribution Data: Detecting out-of-distribution data; Training a robust model in the open world (e.g., open-set learning, out-of-distribution generalization).
Learning/Inference under Distribution Shift (a.k.a., Transfer Learning): Leveraging the knowledge from domains with abundant labels (i.e., source domains)/pre-trained models (i.e., source models) to complete classification/clustering tasks in an unlabeled domain (i.e., target domain), where two domains are different but related.
Protecting Data Privacy: Training a model to ensure that the training data will not be obtained by inverting the model (i.e., defending against model-inversion attacks).
Research Experience
Assistant Professor (May 2022--now)
- The University of Melbourne, Melbourne, Australia
- A tenured teaching and research position in machine learning and data science
Visiting Fellow (May 2022--now)
- Australian Artificial Intelligence Institute, UTS, Sydney, Australia
- Collaborating with Dist. Prof. Jie Lu
Visiting Scientist (July 2021--now)
- Imperfect Information Learning Team,
- RIKEN
Center for Advanced Intelligence Project (RIKEN-AIP), Tokyo, Japan
- Collaborating with Prof. Masashi Sugiyama and Dr. Gang Niu
Australian Laureate Posdoc Researcher (May 2020--May 2021)
- Australian Artificial Intelligence Institute, UTS, Sydney, Australia
- Advisor: Dist. Prof. Jie Lu
- Project: Autonomous Transfer Learning
Visiting Researcher (August 2019--November 2019)
- Gatsby Computational Neuroscience Unit, UCL, London, UK
- Advisor: Prof. Arthur Gretton
- Collaborators: Dr. Danica J. Sutherland, Dr. Wenkai Xu
- Project: Learning Deep Kernels for Two Sample Test
Research Intern (March 2019--July 2019)
- Imperfect Information Learning Team, RIKEN-AIP, Tokyo, Japan
- Advisor: Prof. Masashi Sugiyama
- Collaborators: Dr. Gang Niu and Dr. Bo Han
- Project: Robust Unsupervised Domain Adaptation
|